Geostatistical Modeling plays a crucial role in Static and Dynamic Reservoir Characterization. Building the model is a complex and time-consuming task that involves integrating multiple datasets and feature engineering.
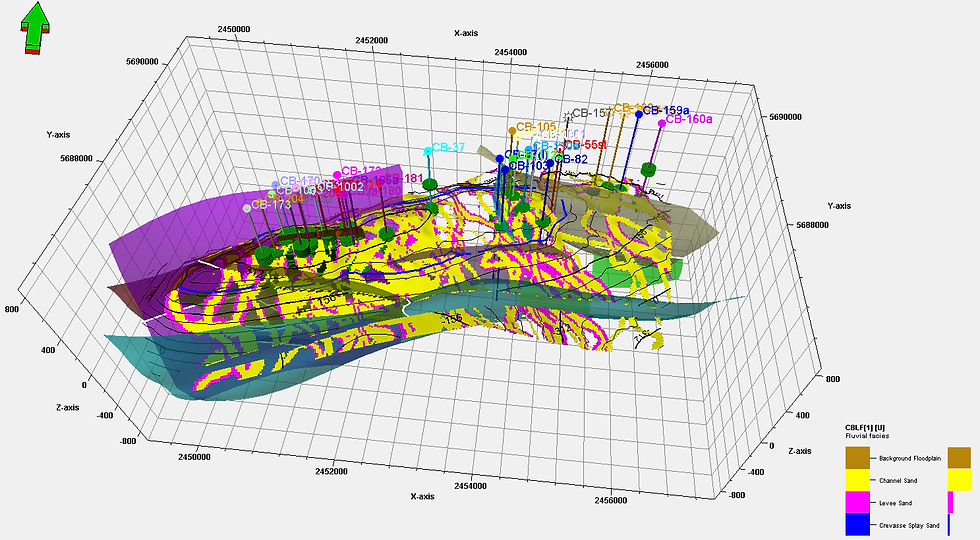
Once the base case of the geostatistical model has been completed, the image above depicts an example, a reliable (usually large) number N of realizations is needed to successfully represent the complexities of the subsurface, its intrinsic heterogeneity and variability, and, most importantly, to assess and quantify the key uncertainties that propagate from inputs throughout the entire model-building process. Therefore, a whole set of N stochastic realizations, not just one, is required to construct a robust and predictive reservoir model. For more information, refer to "Geostatistical Reservoir Modeling" by Clayton Deutsch.
It must be emphasized that the N geostatistical realizations, not a single one, collectively represent the MODEL of the RESERVOIR.
As mentioned in a previous post, to estimate the number N of realizations, a Monte Carlo process is required. This process involves assigning probability distributions to the reservoir variables that contribute the most to model uncertainty. The metric typically selected for this process is the Original Oil In Place or STOIIP.
When building reservoir models, there are deterministic and stochastic approaches. Creating a reliable stochastic model is crucial for accurately predicting reservoir behavior, especially in mature fields.
The STOIIP data is used to create a histogram, and realizations are incrementally added, as shown in the image above, until a bell-shaped histogram is achieved. The latest indicates that the Central Limit Theorem applies. At this stage, the number N of realizations (or more) is considered reliable for representing and quantifying the uncertainty in the Geostatistical Model. In this particular case, there were approximately 3,600 geostatistical realizations.
Again, the full set of N realizations is always needed to make predictions about the reservoir's static and dynamic performance. These realizations are used to evaluate key statistical summaries and in dynamic simulations, p10, p50, and p90 percentile realizations are commonly considered.
It is crucial to follow the previous steps when conducting reservoir modeling, especially for mature fields. It is important to accurately quantify and analyze uncertainties to effectively address the delineation of bypassed oil/gas areas and to correctly evaluate the remaining hydrocarbon volumes.
Before making predictions, it is important to verify the model by using Minimum Acceptance Criteria and any relevant independent data or information available. To illustrate it, an example of a mature oilfield in Tabasco Mexico is considered (refer to the image below). For instance, the findings of a microscopic-scale Geochemical study and the results of interpreting Seismic Inversion Volumes at a macroscopic scale were utilized to validate and cross-check the model.
The figure below shows well (a) and well (b). In both wells, a comparison is made between the synthetic-STOIIP-log (on the right track) evaluated using the Reservoir Model and the presence of Kerogen/Organic Matter reported by the microscopic geochemical analysis (second track, from right to left). There is a remarkable correspondence between the two.
Next, at the bottom of the figure, in well (c), the synthetic STOIIP log (displayed on the right) is compared with the Inversion-Seismic-derived and original well logs. Seismic attributes and AVO were also taken into consideration. A strong correspondence is observed, especially at the top of the interval. The model's predictive capability has been successfully tested at both ends of the scale.
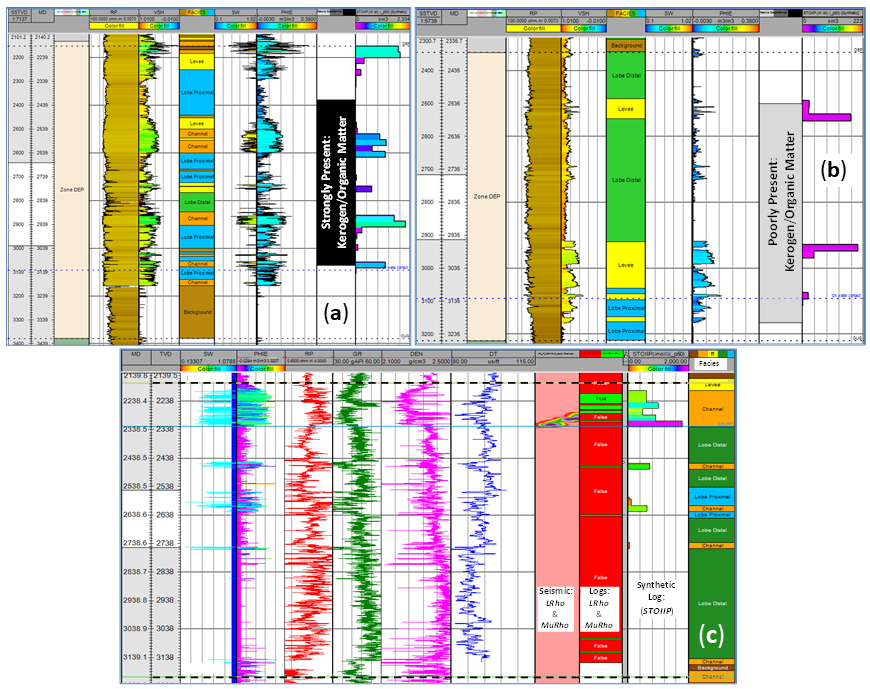
The methods and techniques outlined here can be easily adapted and applied in various other fields of knowledge. Certainly, insights like these can be crucial for allocating resources and optimizing operators' investment budgets.
Would you like to learn more about our services and solutions? Feel free to reach out to us directly or through our partners GeolOil LLC and DataMateDataRobot for prompt and reliable assistance.
Commentaires