In the competitive business landscape, maximizing profitability is a key objective for organizations. One crucial aspect in achieving this goal is understanding the factors that influence order profit. This post explores how regression, a powerful statistical technique, can be leveraged, using the DataRobot AI Platform, to predict order profit, providing valuable insights to data analysts, commercial engineers, and marketers.

Leveraging Regression Modeling in DataRobot AI
This is a challenging NLP use case that involves a comprehensive dataset with over 50,000 records (15.64 MB) from a global retail superstore. The dataset, downloaded from Kaggle, has a usability rating of 10.00. It includes geographical, text, categorical, and numerical data. The task is a regression task aimed at predicting the profit generated from the orders.
Regression analysis is a statistical method used to determine the relationship between a dependent variable (in this case, order profit) and one or more independent variables (order features). By fitting a mathematical model to the data, regression allows us to make predictions about the order profit based on the values of the independent variables.
The image below illustrates a Lift chart, a valuable tool delivered by DataRobot utilized in evaluating the performance of predictive models. Specifically, the chart shown pertains to the regression model designed to predict profit. The Lift chart is a graphical representation that showcases how effective the model is at identifying positive outcomes compared to a random selection. It provides insights into the model's ability to prioritize or rank predictions accurately.
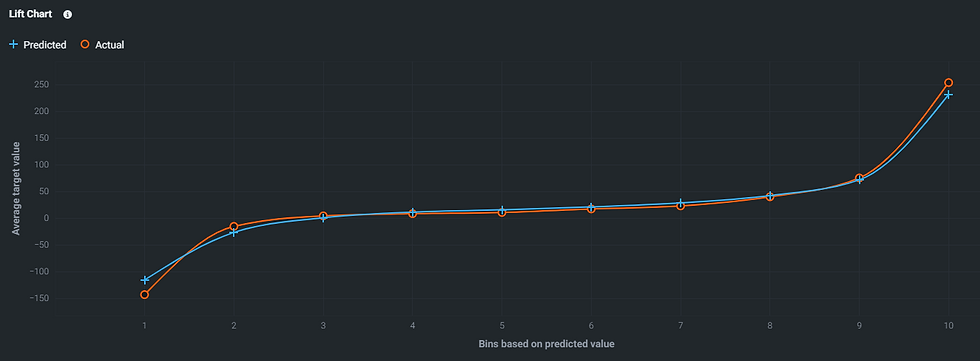
This Lift Chart showcases the accuracy of the profit prediction model. The close alignment of the predicted (blue) and actual (orange) profit lines demonstrates the model's ability to effectively identify which orders are most likely to generate higher profits. This insight empowers retailers to optimize their operations, marketing, and even customer interactions for maximum profitability.
The image below illustrates the DataRobot Feature Impact chart. It's a powerful visualization tool that provides valuable insights into the importance of different features in a machine-learning model. This chart illustrates the impact of each feature on the model's predictions, helping to understand which variables are driving the outcomes.

The Feature Impact chart above highlights the key factors driving profitability in the retail superstore. As expected, the features Sales, Discount, and Shipping Cost are major contributors. Interestingly, Product Name also emerges as a significant factor, suggesting that specific products or brands play a crucial role in driving profits. This insight can be leveraged to optimize product assortment, pricing strategies, and marketing campaigns.
This insight empowers businesses to optimize pricing and discount strategies, carefully manage shipping costs, and potentially adjust product offerings to maximize profitability. The ability to identify and leverage these key drivers gives retailers a competitive edge in a dynamic market.
Below, the Feature Effects chart is depicted. This is commonly employed to identify which features or factors have the most significant influence on the target variable. By plotting the effects of each feature on the outcome (keeping fixed the others), analysts can gain insights into the relationships between variables and make informed decisions based on the patterns observed. The Feature Effects chart often helps in identifying key drivers of a specific result, enabling organizations to focus their efforts on optimizing these factors for improvement of performance, namely, increase the profit.
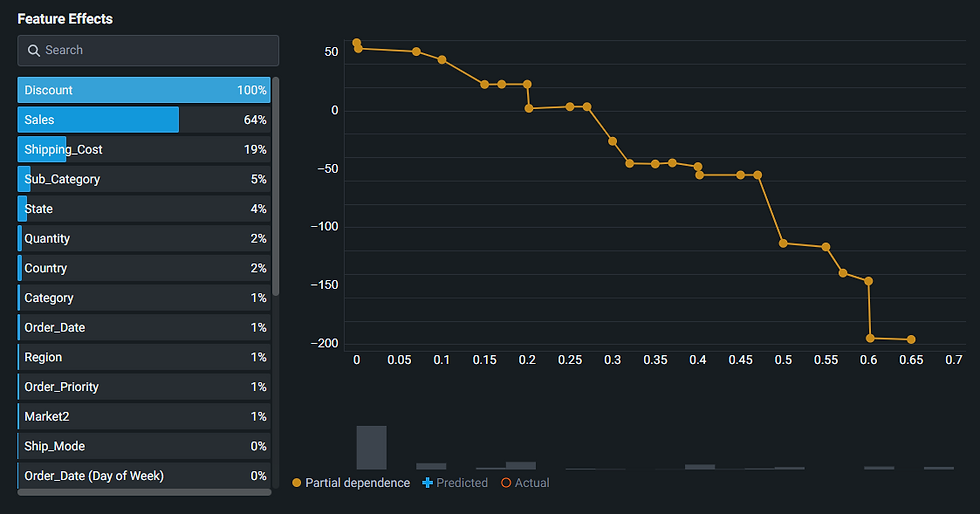
To delve deeper into the evaluation of the model's performance, it is crucial to analyze the residuals. Residuals are the differences between the observed values and the predicted values by the model. By examining the residuals, we can gain valuable insights into how well the model fits the data and identify any patterns or trends that may indicate areas for improvement. The closer the residual points distribution is to the horizontal line, the better the model prediction power.
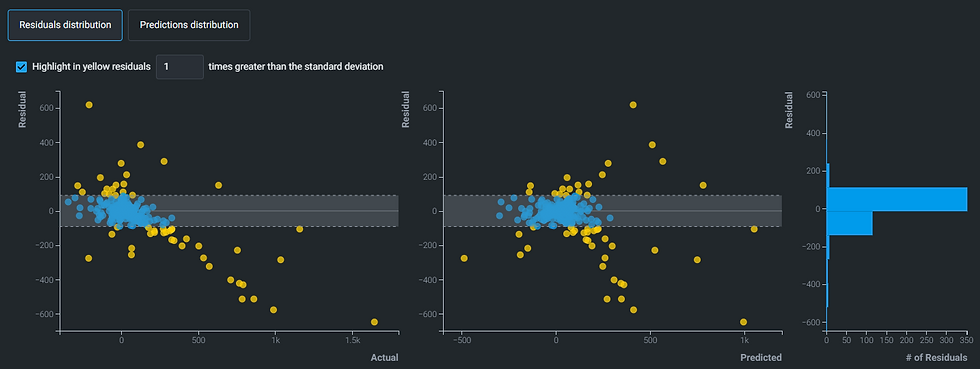
Regarding the image below, when evaluating a model's prediction power, the proximity of the cloud of points to the 45° line serves as a crucial indicator. This line represents perfect predictions, where the actual values match the predicted values. Therefore, the closer the cloud of points aligns with this line, the more accurate and reliable the model's predictions are considered to be.
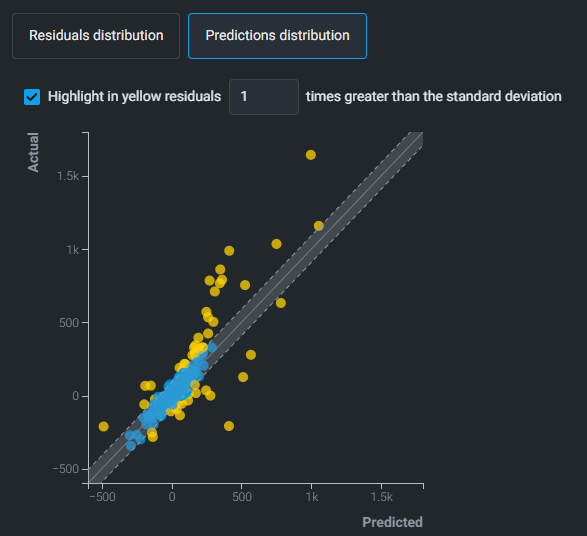
After training the model, predictions were made on a part of the data that was not previously seen. This is where DataRobot's Prediction Explanations come in handy. This functionality aids in comprehending the variables that impact a particular prediction, rather than assessing the model's general performance.
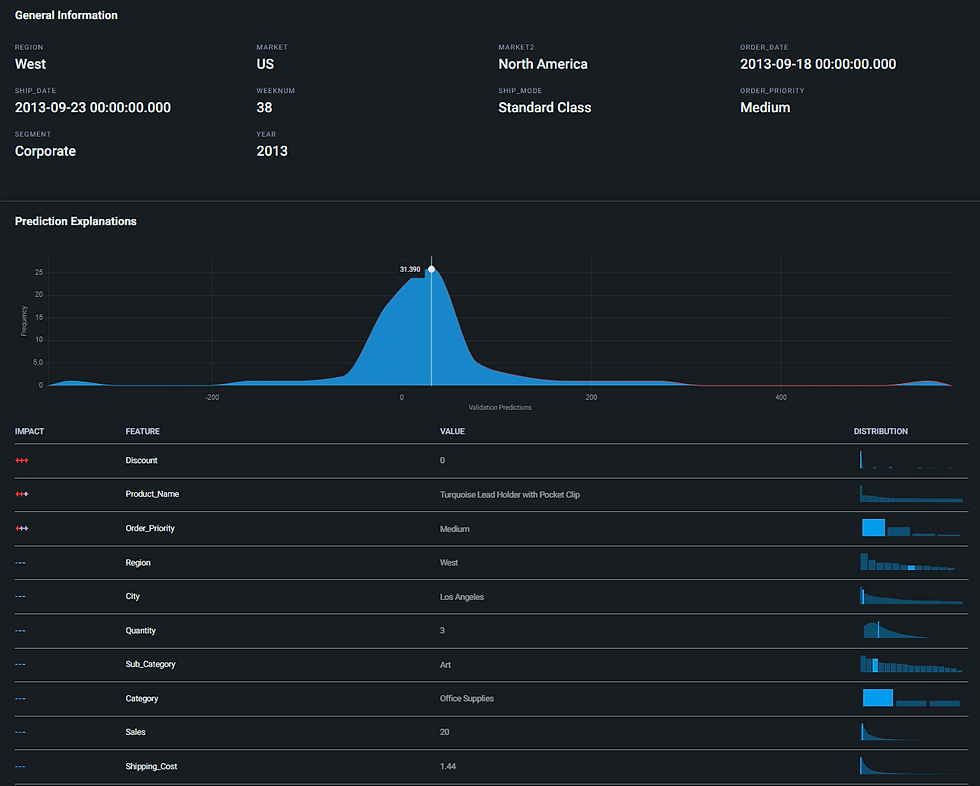
DataRobot not only predicts profit but also explains why those predictions are made. In the image above, we see that a discount of 0% on a "Turquoise Lead Holder" significantly contributed to the predicted profit of $31.90. Understanding these drivers empowers retailers to make data-backed decisions about pricing, promotions, and inventory. Imagine being able to analyze every order with this level of detail - that's the power of DataRobot!
The What-if tool, depicted in the image below, allows users to simulate various scenarios and analyze the potential outcomes, enabling them to anticipate different possibilities and plan accordingly. This feature is particularly useful for scenario planning, risk assessment, and decision-making processes.
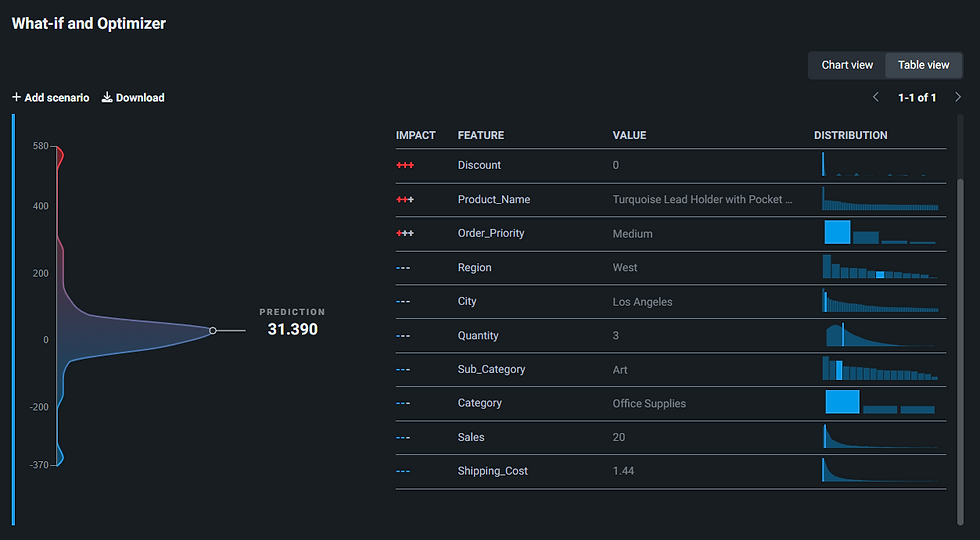
Relevance of Order Profit Prediction
Predicting order profit is of paramount importance for several reasons. It empowers data analysts to identify the key drivers of profitability, enabling them to make informed recommendations to decision-makers. Commercial engineers can utilize these insights to optimize pricing strategies and improve order fulfillment processes. Marketers can leverage this knowledge to fine-tune marketing campaigns, targeting customers likely to generate higher profits.
Conclusion
Utilizing regression provides a strong foundation for forecasting order profit. Leveraging this statistical method with the DataRobot AI Platform enables businesses to acquire important understandings regarding the elements influencing their profitability. Armed with this information, data analysts, business engineers, and marketers are empowered to make informed decisions, enhance efficiencies, and foster the expansion of their companies.
If you want to outperform your competitors, think about using DataRobot AI in your business now! Book a consultation with us to discover the opportunities and acquire more profound insights.
Коментарі