Unveiling the essential elements that make a dress a must-have!
- Angel Solutions (SDG)
- Sep 27, 2024
- 2 min read
Updated: Oct 1, 2024
Have you ever considered what drives a customer to click the coveted "recommend" button? Delving into the world of women's fashion e-commerce, we have uncovered some fascinating insights.
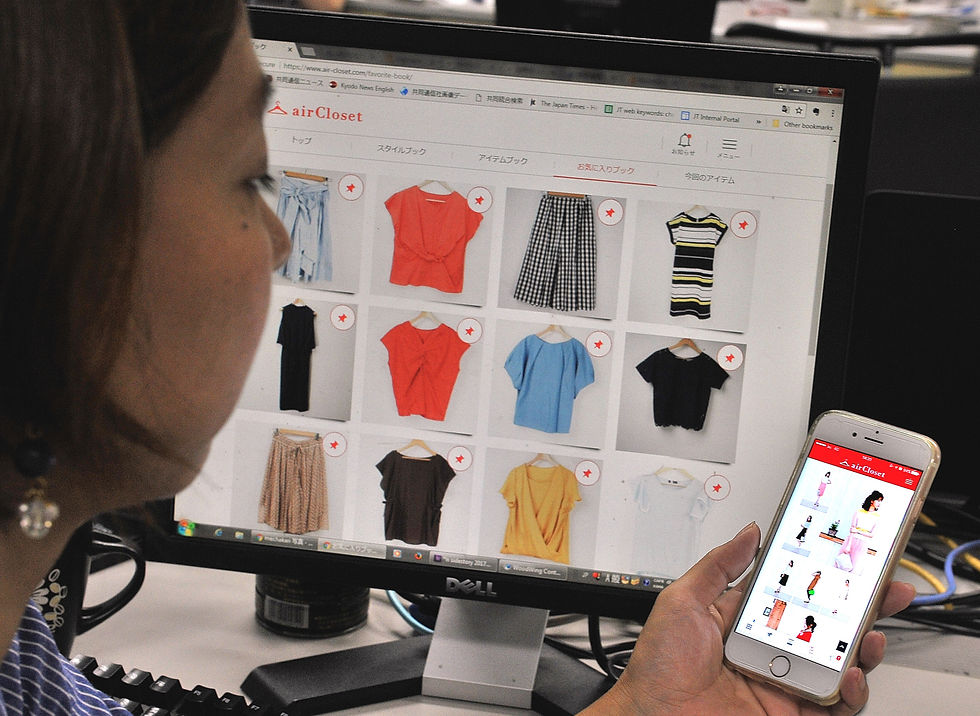
Model overview
This NLP use case is moderately challenging. The dataset comprises more than 20,000 instances sourced from an online retail platform and was obtained from Kaggle (rated usability: 10.0). It encompasses text, categorical, and numerical data. The objective is to perform a binary classification task, predicting whether the buyer will recommend the product they purchased. The model was created and executed using the DataRobot AI platform.
Model's metrics analysis
We now offer a summary of the model's metrics analysis in a Google Sheet for a brief overview:
Overall, the analyses reveal a well-performing model with strong reliance on textual data. However, potential class imbalance, nuanced feature interactions, and the risk of bias require further attention to ensure optimal performance, fairness, and interpretability.
First things first, words matter
Our analysis shows that customer reviews and the names of products are key factors in predicting recommendations. It is not just about positive or negative feedback - the specific language used gives a comprehensive portrayal of the apparel. A product labeled as "lovely" and "perfectly fitting" emerges as a preferred option, while phrases like "low-quality material" raise red flags.
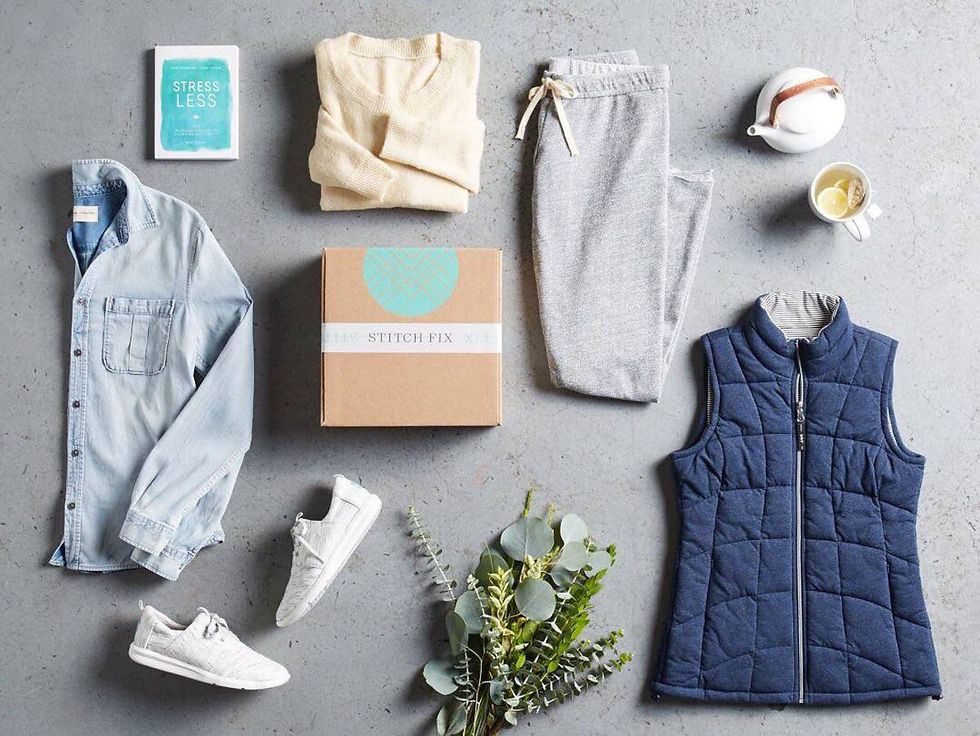
Interestingly the number of positive feedback counts plays a role...
The relationship is not linear. A couple of positive reviews can have a significant impact, but the effect levels off at a certain stage. Authenticity appears to be more important than simply having a large number of reviews.
Furthermore, we observed an intriguing interaction with the age of the buyer. Although not the most dominant factor, it does have a subtle influence on predictions. The model acknowledges that different age brackets may have different preferences and expectations.
What's the takeaway for retailers?
Focus on those product descriptions! Clear, evocative language highlighting the garment's best features is key.
Encourage genuine reviews from customers, but don't obsess over massive numbers.
And remember, understanding your target demographic is crucial: a dress that's "perfect" for one age group might not be the same for another.
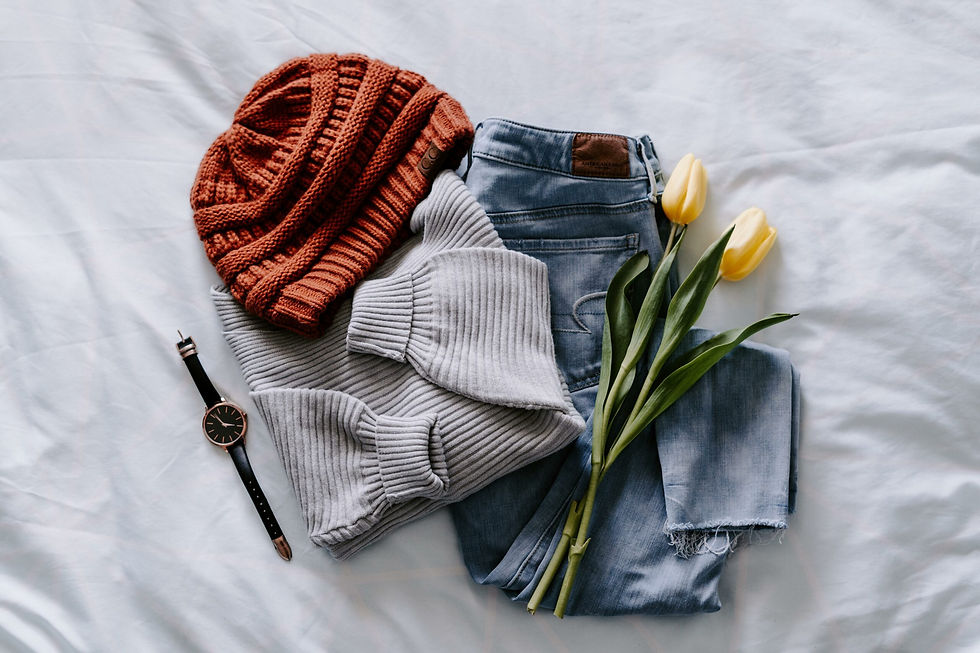
Ensuring caution is crucial when working with data, given its susceptibility to bias despite its potency. Maintaining fairness and inclusivity in our models is paramount. To prevent the perpetuation of stereotypes, it is essential to engage a varied group of reviewers and products consistently.

The realm of fashion is intricate and constantly changing, yet AutoML is aiding us in unraveling its mysteries. By grasping the factors behind suggestions, we can enhance the shopping experience for each individual through a more tailored and fulfilling approach.
Would you like to learn more about our services and solutions? Feel free to reach out to us for prompt and reliable assistance.
Comments